Understanding Machine Learning: A Comprehensive Guide
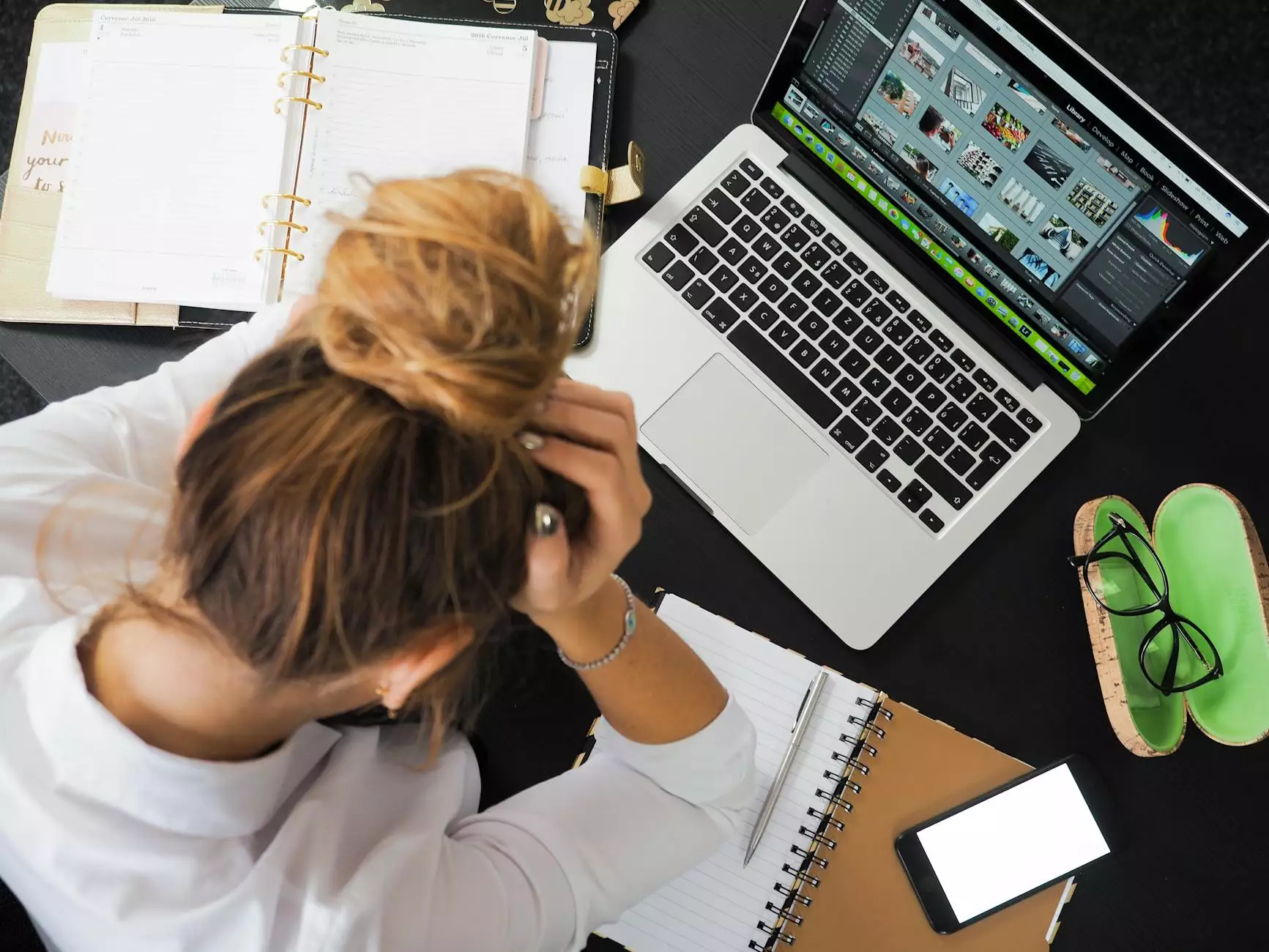
Machine learning has emerged as one of the most transformative technologies in the modern business landscape. By leveraging vast amounts of data and advanced algorithms, organizations are now able to make more informed decisions, optimize processes, and enhance customer experiences. This article delves deep into the world of machine learning, highlighting its significance in business and providing actionable insights for its implementation.
What is Machine Learning?
At its core, machine learning is a subset of artificial intelligence (AI) that enables systems to learn from data, identify patterns, and make predictions without being explicitly programmed. The essence of machine learning lies in its ability to improve its performance over time as it processes more data. This makes it a vital tool for modern businesses that rely on data-driven decision-making.
The Importance of Machine Learning in Business
In today’s fast-paced digital world, businesses are inundated with data from various sources. Utilizing machine learning allows organizations to harness this data effectively. Here are some compelling reasons why machine learning is indispensable in the business landscape:
- Enhanced Decision-Making: Machine learning algorithms analyze vast datasets quickly, providing businesses with actionable insights that facilitate better decision-making.
- Operational Efficiency: By automating routine tasks, machine learning reduces operational costs and increases efficiency, allowing employees to focus on higher-value tasks.
- Personalization: Businesses can leverage machine learning to analyze customer behavior, enabling them to offer personalized services and products, thus enhancing customer satisfaction and loyalty.
- Predictive Analytics: Machine learning models can predict future trends based on historical data, allowing companies to be proactive rather than reactive.
Applications of Machine Learning in Different Industries
The versatility of machine learning transcends various industries. Here, we explore how different sectors are integrating machine learning into their operations:
1. Healthcare
In the healthcare industry, machine learning plays a crucial role in patient care and operational efficiency. Its applications include:
- Disease Diagnosis: Algorithms can analyze medical images and patient data to assist doctors in diagnosing diseases more accurately and swiftly.
- Predictive Medicine: Machine learning can predict patient outcomes by analyzing patterns in treatment data, helping in personalized treatment plans.
- Operational Optimization: Hospitals utilize machine learning to manage resource allocation, ensuring that medical staff and facilities are well distributed to meet patient needs.
2. Finance
The finance sector has embraced machine learning for various purposes, including:
- Fraud Detection: Financial institutions use machine learning models to detect unusual patterns that may indicate fraudulent activities.
- Risk Management: By analyzing historical data, machine learning helps organizations assess risk levels associated with loans and investments.
- Algorithmic Trading: Machine learning algorithms process vast amounts of market data in real-time to make trading decisions swiftly and effectively.
3. Retail
In the retail industry, machine learning enhances customer engagement and operational efficiency:
- Inventory Management: Predictive analytics model helped retailers optimize inventory levels based on the anticipated demand.
- Customer Segmentation: Machine learning algorithms analyze purchase history to identify distinct customer segments for targeted marketing strategies.
- Recommendation Systems: E-commerce platforms utilize machine learning to recommend products based on previous customer behavior, increasing sales and customer satisfaction.
Challenges in Implementing Machine Learning Solutions
While the benefits of machine learning are significant, there are also challenges that businesses need to address:
1. Data Quality
The effectiveness of machine learning algorithms heavily depends on the quality of the input data. Businesses must ensure that the data is clean, relevant, and representative of the problem they are trying to solve.
2. Talent Shortage
There is a notable shortage of skilled professionals specializing in machine learning and data science. Organizations often struggle to find the right talent to develop and implement machine learning solutions.
3. Integration with Existing Systems
Integrating machine learning solutions with existing IT infrastructure can be complex. Businesses need to consider compatibility and scalability concerns to ensure seamless operations.
Steps to Successfully Implement Machine Learning in Your Business
For businesses looking to integrate machine learning, here are key steps to consider:
- Define Your Goals: Clearly establish what you aim to achieve with machine learning. Whether it’s improving customer experience or optimizing operations, having a clear goal is essential.
- Data Collection: Gather relevant data from various sources and ensure that it is of high quality and quantity. This is crucial for training effective machine learning models.
- Choose the Right Tools: There are numerous machine learning frameworks available, such as TensorFlow, PyTorch, and Scikit-learn. Selecting the right tools that align with your business goals is vital.
- Develop the Model: Start with small, manageable projects to develop and test machine learning models. This iterative process allows for improvements based on feedback.
- Monitor and Evaluate: Continuously monitor the performance of the machine learning models and adjust them as necessary. Evaluation metrics are crucial to gauge success and areas for improvement.
Future Trends in Machine Learning
The future of machine learning is bright, with several trends expected to shape its evolution:
1. Automated Machine Learning (AutoML)
AutoML aims to simplify the machine learning process, allowing individuals with minimal data science expertise to build models effectively. This will democratize machine learning and expand its adoption across industries.
2. Explainable AI
As businesses increasingly rely on machine learning for critical decisions, the need for transparency and explainability is becoming paramount. Techniques that make machine learning models more interpretable will gain traction.
3. Edge Computing
With the rise of IoT devices, machine learning models will increasingly be deployed at the edge, enabling quick decision-making locally without needing to send data to the cloud.
4. Integration of AI and IoT
The combination of AI and IoT will create smarter systems that can analyze real-time data from connected devices, leading to smarter decision-making in various applications, from smart homes to industrial automation.
Conclusion
In summary, machine learning offers unparalleled opportunities for businesses to innovate, enhance efficiency, and improve customer experiences. Despite the challenges, the potential of machine learning in transforming operations is undeniable. By understanding its applications, overcoming implementation hurdles, and staying informed about future trends, businesses can position themselves at the forefront of their industries. The journey towards integrating machine learning may be complex, but the rewards are well worth the effort.
machine learning article